Case Studies
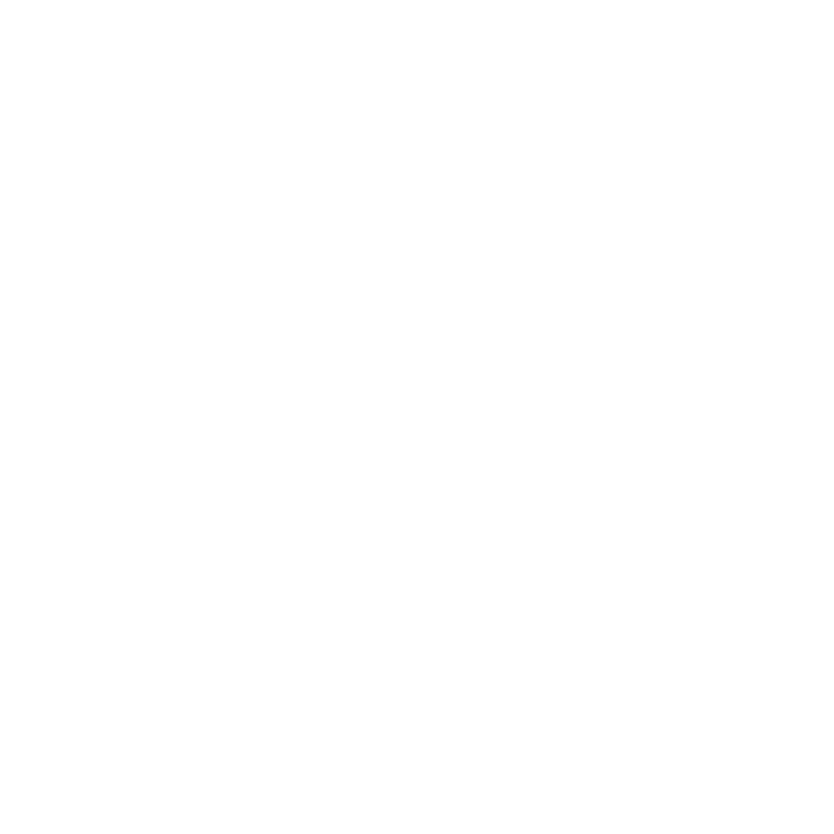
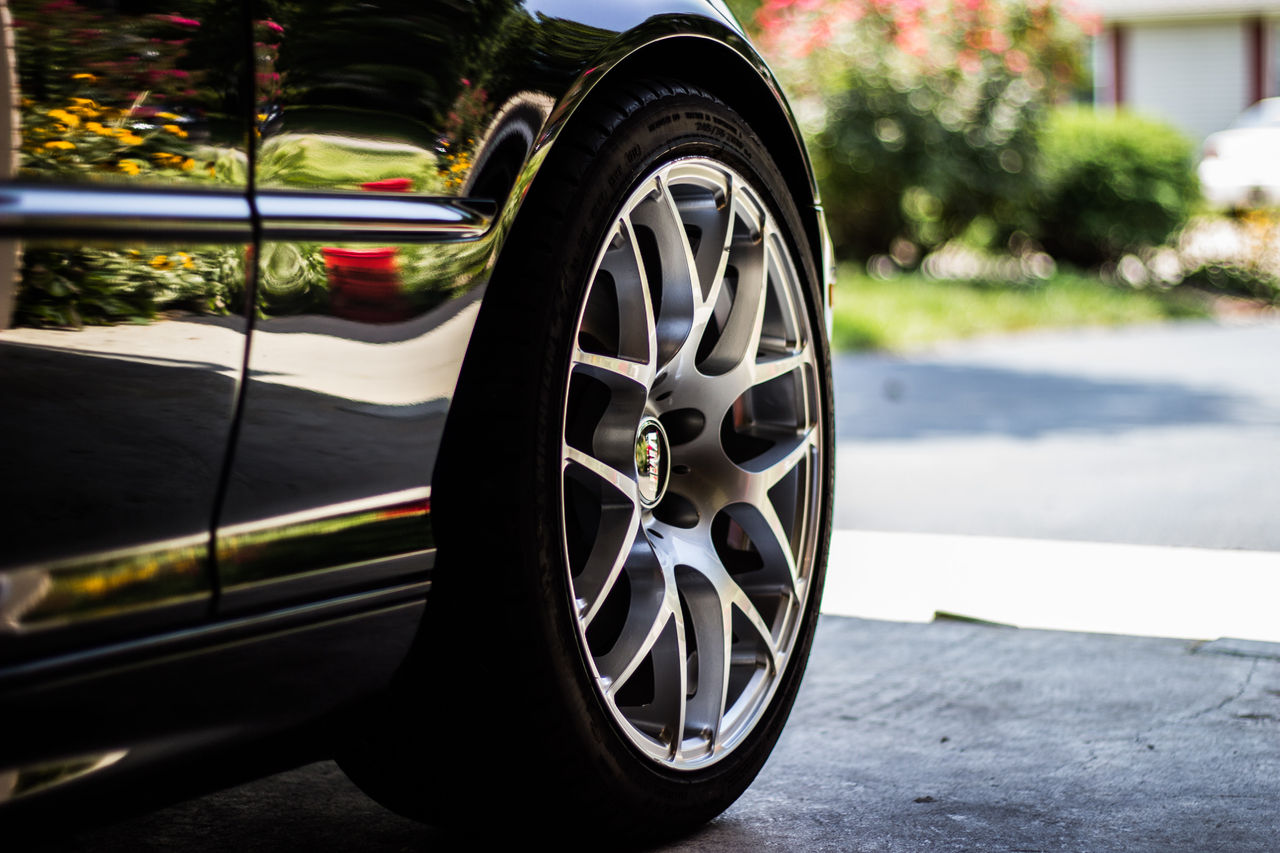
Featured Case Study
How Maxion Wheels Increased Continuous Improvement Cost Savings by 19% With Minitab Engage
Maxion Wheels, the world's largest wheel producer, increased annual cost savings by over 19% by incorporating Minitab Engage® into their project management system. Engage’s infrastructure allowed for instant visibility and intelligible metrics across 1,600 projects and 1,500 users, resulting in improved productivity and collaboration.
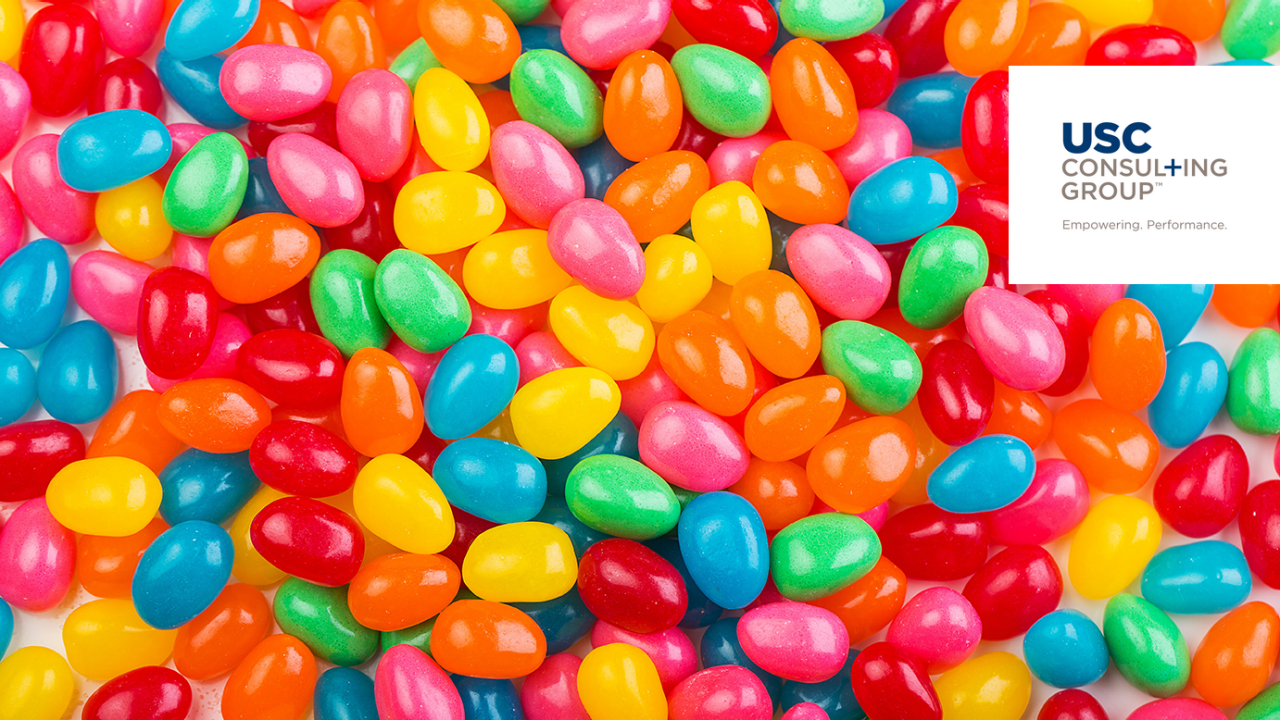
Featured Case Study
How USC Consulting Group Reduced Overfill and Saved a Leading Candy Manufacturer $1.2M with the Help of Minitab Statistical Software
For over 50 years, USC Consulting Group (USCCG) has been driving operational excellence with a mission to provide real value by improving financial performance. USCCG helps companies reach their highest potential by improving processes and operations across their entire supply chain.
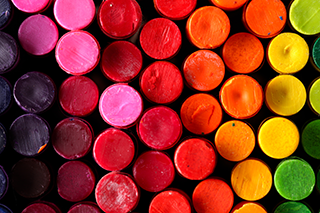
Featured Case Study
The Color of Quality: How Crayola Uses Data to Deliver the Perfect Crayon
Analyzing and acting on data transformed the way Crayola does business and has enabled the company to concentrate resources where they'll have the biggest impact.
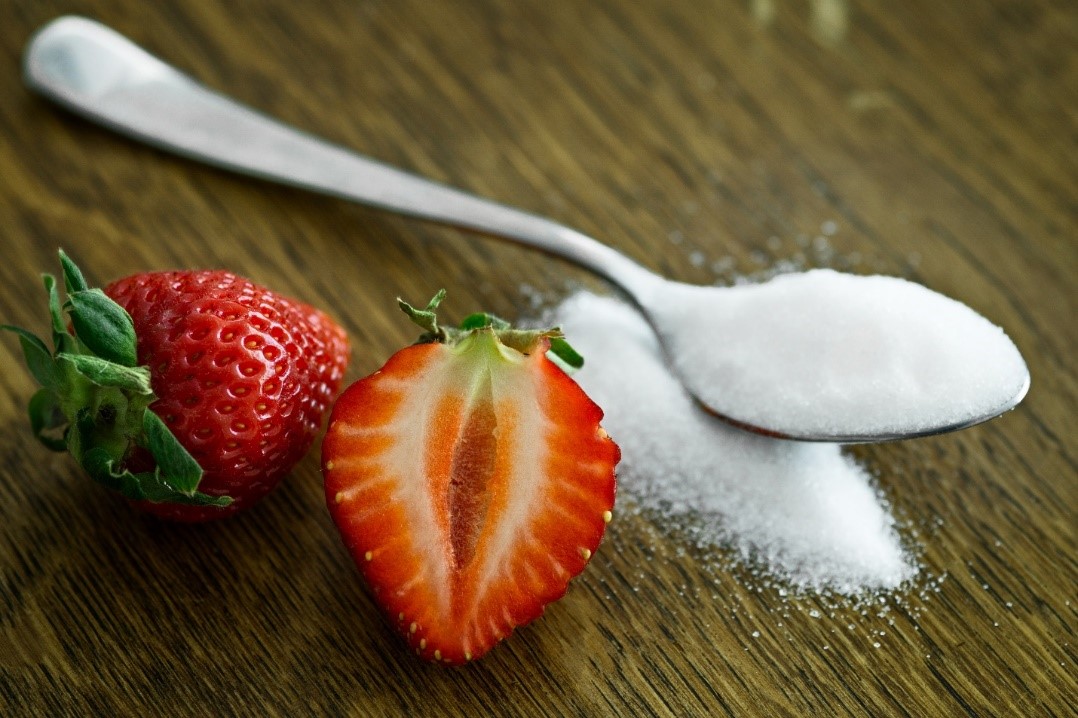
Featured Case Study
Helping Tate & Lyle Ensure Sweeteners Feel More Like Plain Sugar
When Tate & Lyle faced a challenge in the process of refining corn sugars, they turned to Minitab software for assistance.
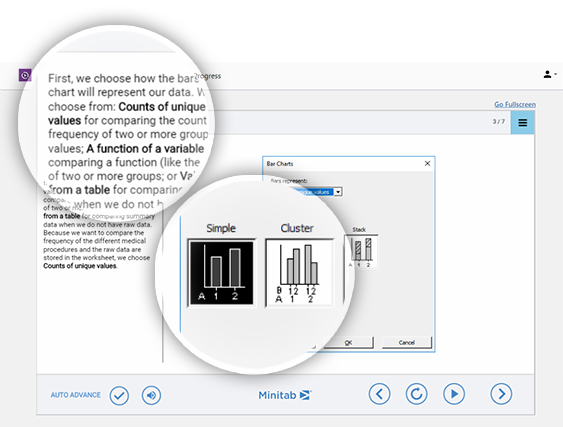
Featured Case Study
Getting Trainees Up to Speed with Stats: Cummins Trusts Quality Trainer
The diverse range of statistical knowledge among Cummins Inc.'s employees made teaching new Six Sigma trainees a challenge. Then the company tried Quality Trainer by Minitab.
- Automotive
- Energy and Resources
- Finance
- Government
- Healthcare
- Manufacturing
- Medical Devices
- Mining
- Quality Improvement
- Service
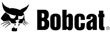
Bobcat
A quality expert at Bobcat used Minitab Statistical Software to increase the speed of a laser-cutting process while improving part quality, for a potential impact of more than $1 million.
.png)
Ford Motor Company DOE
Faced with the launch of the Ford Fiesta, Ford Motor Company trusted Minitab for the statistical tools necessary to deliver a quality product and a successful introduction.
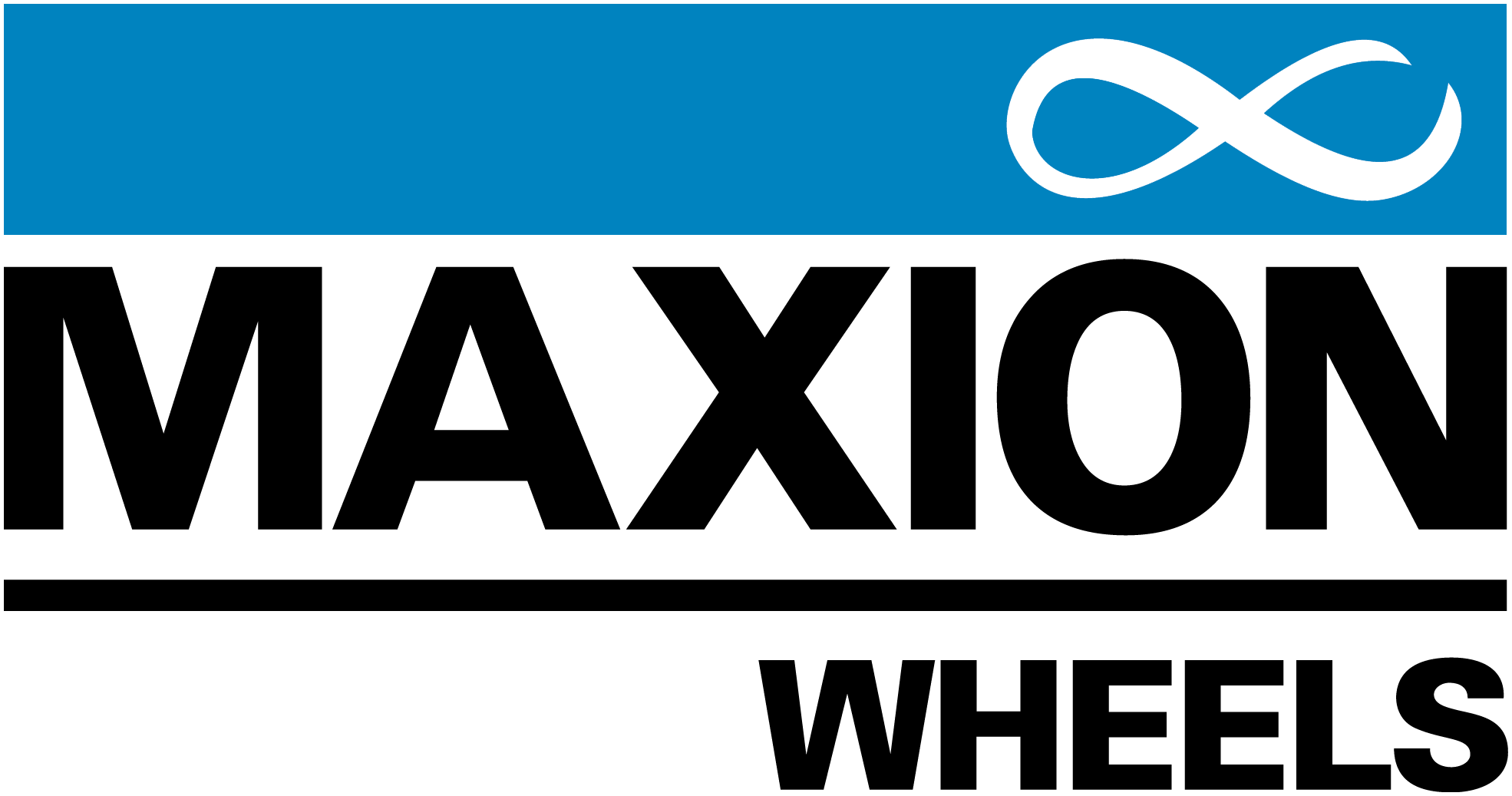
Maxion Wheels
Maxion Wheels, the world's largest wheel producer, sought immediate visibility into their continuous improvement and operational excellence projects. They chose to implement Minitab Engage, which provided workflow functions that enabled stakeholders to easily track and report on progress with data visible to administrators. As a result, the company improved visibility, saved time and achieved cost savings.
Global Solar Technology and Renewable Energy Provider
One of the world’s largest solar technology and renewable energy companies needed to control the thickness of its silicon nitride film to improve energy efficiency levels. Learn how the company was able to pinpoint and rectify the causes for the differences in film thickness using the powerful charting and analysis tools included in Minitab® Statistical Software and Minitab Engage®.
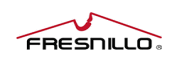
Fresnillo Plc
Fresnillo Plc increased its monthly revenue by over $2 million using Lean Six Sigma methodologies and Minitab Statistical Software to examine the silver recovery process at its Saucito mine.
.png)
Mosaic Corporation
Find out how a Six Sigma team at Mosaic Corporation, armed with an excellent knowledge of process improvement and powerful tools including Minitab Statistical Software, increased productivity by more than 50 percent—and made a financial impact of more than $12 million per year.
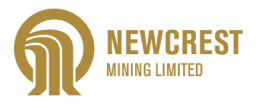
Newcrest Mining Ltd
With Minitab Statistical Software, Newcrest Mining can get an additional load of ore per truck each day from its Cadia Hill mine.
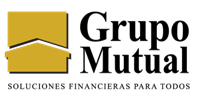
Grupo Mutual
A project team at Grupo Mutual, one of Costa Rica’s leading financial institutions sought to improve the cash flow among 55 of its bank branches. Using Lean Six Sigma methods and Minitab Statistical Software enabled the team to increase revenue by $1.1 million.

Edward Jones
With the current growth of the financial firm—and plans for future expansion—Edward Jones wanted to ensure its world-class service continues to exceed customers’ expectations. Armed with Lean Six Sigma tactics and the statistical tools in Minitab Statistical Software, the business process improvement team at Edward Jones set out to assess how the firm could continue to meet its high customer service standards in the midst of increasing service demands.
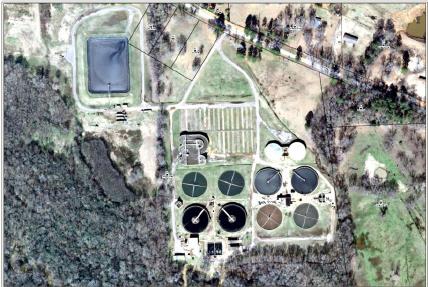
City of Tyler Texas
The City of Tyler, Texas has saved more than $5 million through Lean Six Sigma projects. In one such project, city employees used Minitab Statistical Software to show that using less magnesium hydroxide to treat wastewater still ensured compliance with state regulations—while shaving some $80,000 in expenses.
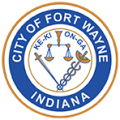
City of Fort Wayne Indiana
Indiana’s second-largest city used Minitab and Six Sigma to improve municipal services—and save millions of dollars.
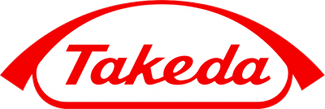
Takeda Boosts Drug Production with the Help of Machine Learning Models
When Takeda Pharmaceutical Company Ltd. wanted to produce more of its three blood clotting drugs more efficiently, it used Minitab Statistical Software's machine learning features to identify a way to increase yield from the cell cultures used in production.
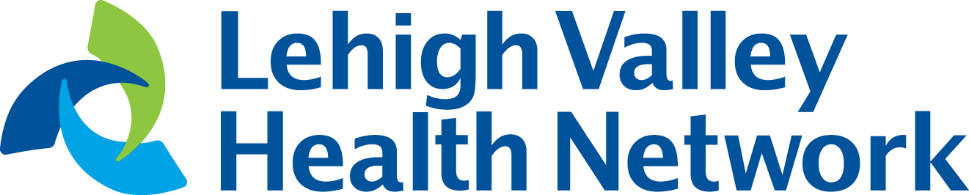
Lehigh Valley Health Network
Lehigh Valley Health Network (LVHN) has achieved Quality Milestones throughout its 100+ year history. Its quality journey has recently added Lean Six Sigma tools and Minitab statistical analysis software to help achieve improvements with patient-centered care.

Connections Health Solutions
Connections Health Solutions was asked to assume management of the Tucson, Arizona Crisis Response Center in April 2014 due to quality and safety concerns. Drawing upon their years of experience operating a larger crisis facility in Phoenix, Connections used Lean Six Sigma methods to redesign processes for evaluating, admitting and treating patients. Using Minitab Statistical Software, the Connections team was able to measure their success, which included significant improvements in the timeliness, quality of care, and patient experience overall.
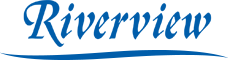
Riverview Hospital Association
With Minitab Statistical Software, the Riverview Hospital Association Lean Six Sigma team was able to perform data analysis to identify patient groups who were scoring lower on patient satisfaction survey questions. This allowed the team to target process improvement efforts to specific patient populations.
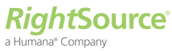
RightSource
When RightSource pharmacy implemented a new prescription management system, its productivity dropped and orders began backing up. Implementing a Lean Six Sigma project using Minitab allowed the company to reduce work-in-progress inventory by 40% and save money at every step.
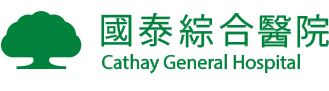
Cathay General Hospital
During an assessment of its angioplasty process for patients suffering from heart attacks, Cathay General Hospital in Taipei, Taiwan used Minitab Statistical Software to analyze data that would help save lives. The tools provided by Minitab allowed healthcare professionals to confidently introduce new treatment options that led to a decrease in the patients’ hospital stay and an increased savings in medical resources.
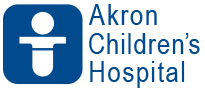
Akron Childrens Hospital Home Care
Akron Children’s Hospital examined its Home Care billing process using Lean Six Sigma methodologies and Minitab Statistical Software. Data analysis enabled both homebound and ambulatory patients to receive quality medical care, and decreased the number of days from delivery to billing by 90%.
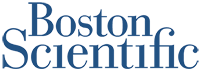
Boston Scientific
During Boston Scientific’s annual assessment of their materials and processes, the medical device manufacturer used Minitab Statistical Software to analyze data that would validate the pouch-sealing process used to package guidewires. The tools provided by Minitab allowed engineers to confidently move forward with the introduction of a new pouch that streamlined packaging across several departments of the corporation and led to a substantial reduction in material costs.
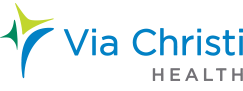
Via Christi Health
With the launch of Via Christi’s Center for Clinical Excellence, the healthcare provider created a team of 5 people, all with Lean and Six Sigma training, to complete projects to make patient care more consistent and its operations more efficient. To perform the data analysis necessary to complete the various projects, Via Christi process improvement teams rely on Minitab Statistical Software.
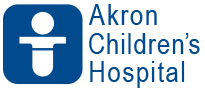
Akron Childrens Hospital Center Extubations
The largest pediatric provider in northeast Ohio used Minitab to analyze their Lean Six Sigma project data, which helped them to decrease their rate of unplanned breathing tube removals for infants in the neonatal intensive care unit—preventing patient setbacks and reducing length of stay.
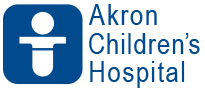
Akron Childrens Hospital
The largest pediatric health care provider in northeast Ohio uses quality techniques pioneered in industry to reduce patient waiting times and make many other improvements. And they trust Minitab Statistical Software to help them analyze their data and understand the results.
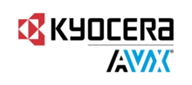
KYOCERA AVX Brings the Voice of the Customer to the Forefront
KYOCERA AVX leveraged Minitab Engage to gain visibility into the quality management systems and continuous improvement programs across its manufacturing plants around the world. As a result, collaboration increased, new learning opportunities appeared, and customers were serviced faster and more efficiently.
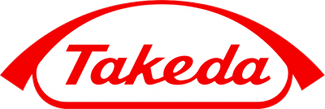
Takeda Boosts Drug Production with the Help of Machine Learning Models
When Takeda Pharmaceutical Company Ltd. wanted to produce more of its three blood clotting drugs more efficiently, it used Minitab Statistical Software's machine learning features to identify a way to increase yield from the cell cultures used in production.
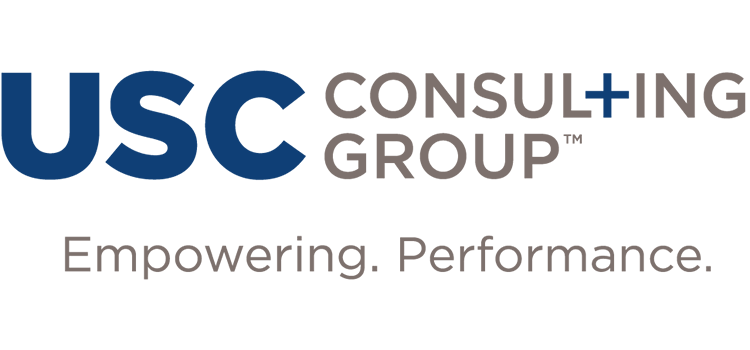
USC Consulting Group
For over 50 years, USC Consulting Group (USCCG) has been driving operational excellence with a mission to provide real value by improving financial performance. USCCG helps companies reach their highest potential by improving processes and operations across their entire supply chain.

Hexpol
The experts at HEXPOL Compounding, a world leader in rubber compounding, pride themselves on sharing their market knowledge and innovation to provide customers in several industries – from auto to construction to healthcare to energy, oil and gas – the right solutions for their applications.

Tate & Lyle
Tate & Lyle is a global leader in the food and beverage industry, validated by a long and impressive track record of “making food extraordinary” by turning corn, tapioca and other raw materials into ingredients that add taste, texture and nutrients to foods. One of their most recognizable products in the US is the sweetener SPLENDA® Sucralose. When they faced a challenge in the process of refining corn sugars, Tate & Lyle turned to Minitab software for assistance.
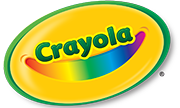
Crayola
Analyzing and acting on data transformed the way Crayola does business and has enabled the company to concentrate resources where they'll have the biggest impact.
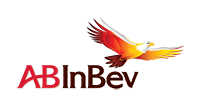
Anheuser-Busch InBev
Anheuser-Busch InBev reduced production line downtime for switching beer brands by 34% and saved more than $430,000.
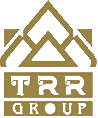
Thai Roong Ruang Sugar Group
Thailand’s leading sugar exporter, Thai Roong Ruang Sugar Group, used Minitab Statistical Software to evaluate and standardize its employee review process in order to address potential bias among managers. Using data analysis, the team established a fair compensation plan that avoids unfair promotions.
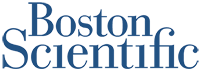
Boston Scientific
During Boston Scientific’s annual assessment of their materials and processes, the medical device manufacturer used Minitab Statistical Software to analyze data that would validate the pouch-sealing process used to package guidewires. The tools provided by Minitab allowed engineers to confidently move forward with the introduction of a new pouch that streamlined packaging across several departments of the corporation and led to a substantial reduction in material costs.

Medtronic
Medtronic’s Black Belt candidates come from many different educational backgrounds, which made training difficult. To make sure candidates shared a common understanding of statistical methods and maximize their potential to succeed, Medtronic turned to Quality Trainer, Minitab’s e-learning course on statistics for quality.
.png)
Ford Motor Company DOE
Faced with the launch of the Ford Fiesta, Ford Motor Company trusted Minitab for the statistical tools necessary to deliver a quality product and a successful introduction.

Cummins
The diverse range of statistical knowledge among Cummins Inc.'s employees made teaching new Six Sigma trainees a challenge. Then the company tried Quality Trainer by Minitab, an e-learning course that teaches Green Belts, Black Belts and other quality professionals the statistics they need. Integrating Quality Trainer into their training program has helped Cummins roll out Six Sigma to more employees.
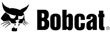
Bobcat
A quality expert at Bobcat used Minitab Statistical Software to increase the speed of a laser-cutting process while improving part quality, for a potential impact of more than $1 million.
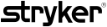
Stryker
Stryker, a leading medical technology company, credits their success to their culture of constant improvement. To make sure employees have the statistical knowledge they need, the company trusts Quality Trainer by Minitab, an e-learning course that teaches quality statistics and how to analyze data with Minitab Statistical Software.
.png)
OneSteel
When the amount of “mill scale” on steel rod from OneSteel’s Laverton Rod Mill began affecting yield and profitability at the company’s wire mill, the company used Six Sigma and the power of Minitab Statistical Software to find solutions—and demonstrate their success.
.png)
Mosaic Corporation
Find out how a Six Sigma team at Mosaic Corporation, armed with an excellent knowledge of process improvement and powerful tools including Minitab Statistical Software, increased productivity by more than 50 percent—and made a financial impact of more than $12 million per year.
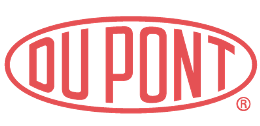
DuPont
DuPont Building Innovations reduced the amount of landfill waste they generated from 81 million pounds per year to zero. The global Six Sigma team that achieved this goal used Minitab Statistical Software to analyze and make sense of the tremendous amount of data gathered throughout the project.
Semiconductor Manufacturing
A semiconductor plant in Thailand used Minitab Statistical Software to accurately forecast electricity consumption and maximize profits. By using data analysis, the plant was able to create a budget that aligned the plant’s energy usage with its production goals.
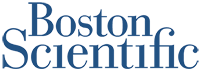
Boston Scientific
During Boston Scientific’s annual assessment of their materials and processes, the medical device manufacturer used Minitab Statistical Software to analyze data that would validate the pouch-sealing process used to package guidewires. The tools provided by Minitab allowed engineers to confidently move forward with the introduction of a new pouch that streamlined packaging across several departments of the corporation and led to a substantial reduction in material costs.

Medtronic
Medtronic’s Black Belt candidates come from many different educational backgrounds, which made training difficult. To make sure candidates shared a common understanding of statistical methods and maximize their potential to succeed, Medtronic turned to Quality Trainer, Minitab’s e-learning course on statistics for quality.
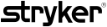
Stryker
Stryker, a leading medical technology company, credits their success to their culture of constant improvement. To make sure employees have the statistical knowledge they need, the company trusts Quality Trainer by Minitab, an e-learning course that teaches quality statistics and how to analyze data with Minitab Statistical Software.
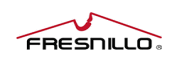
Fresnillo Plc
Fresnillo Plc increased its monthly revenue by over $2 million using Lean Six Sigma methodologies and Minitab Statistical Software to examine the silver recovery process at its Saucito mine.
.png)
Mosaic Corporation
Find out how a Six Sigma team at Mosaic Corporation, armed with an excellent knowledge of process improvement and powerful tools including Minitab Statistical Software, increased productivity by more than 50 percent—and made a financial impact of more than $12 million per year.
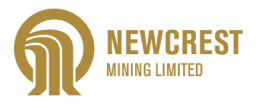
Newcrest Mining Ltd
With Minitab Statistical Software, Newcrest Mining can get an additional load of ore per truck each day from its Cadia Hill mine.
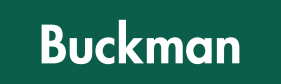
Buckman
Global chemical company Buckman transformed its business by training technical sales associates to use data analysis and Minitab to help customers improve their processes, provide more value to customers, and drive sales—impacting the bottom line.
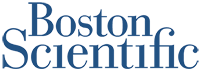
Boston Scientific
During Boston Scientific’s annual assessment of their materials and processes, the medical device manufacturer used Minitab Statistical Software to analyze data that would validate the pouch-sealing process used to package guidewires. The tools provided by Minitab allowed engineers to confidently move forward with the introduction of a new pouch that streamlined packaging across several departments of the corporation and led to a substantial reduction in material costs.
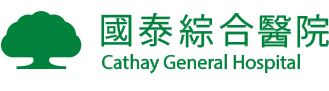
Cathay General Hospital
During an assessment of its angioplasty process for patients suffering from heart attacks, Cathay General Hospital in Taipei, Taiwan used Minitab Statistical Software to analyze data that would help save lives. The tools provided by Minitab allowed healthcare professionals to confidently introduce new treatment options that led to a decrease in the patients’ hospital stay and an increased savings in medical resources.
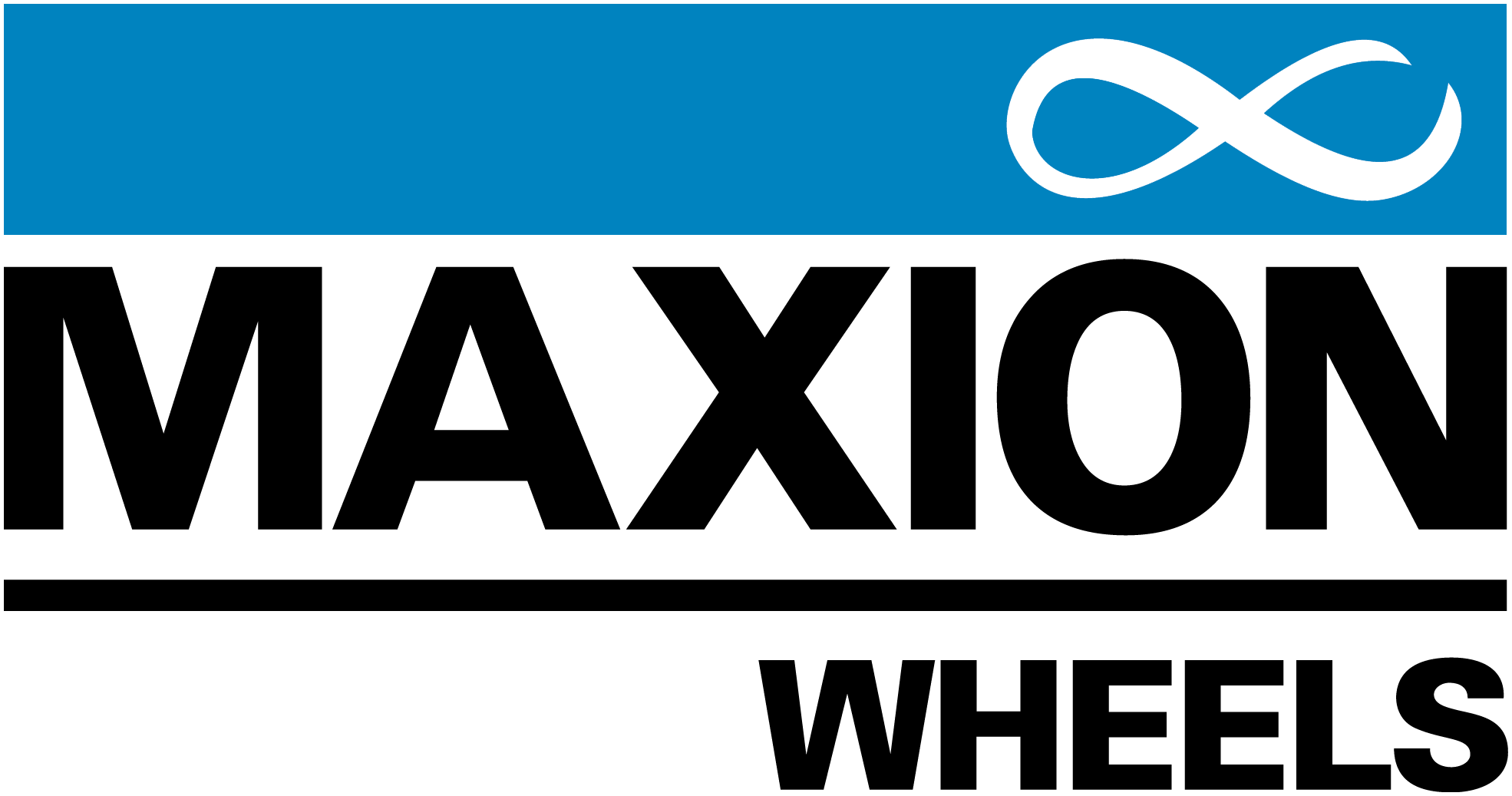
Maxion Wheels
Maxion Wheels, the world's largest wheel producer, sought immediate visibility into their continuous improvement and operational excellence projects. They chose to implement Minitab Engage, which provided workflow functions that enabled stakeholders to easily track and report on progress with data visible to administrators. As a result, the company improved visibility, saved time and achieved cost savings.

Medtronic
Medtronic’s Black Belt candidates come from many different educational backgrounds, which made training difficult. To make sure candidates shared a common understanding of statistical methods and maximize their potential to succeed, Medtronic turned to Quality Trainer, Minitab’s e-learning course on statistics for quality.
.png)
Ford Motor Company DOE
Faced with the launch of the Ford Fiesta, Ford Motor Company trusted Minitab for the statistical tools necessary to deliver a quality product and a successful introduction.
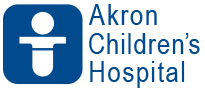
Akron Childrens Hospital Center Extubations
The largest pediatric provider in northeast Ohio used Minitab to analyze their Lean Six Sigma project data, which helped them to decrease their rate of unplanned breathing tube removals for infants in the neonatal intensive care unit—preventing patient setbacks and reducing length of stay.

Cummins
The diverse range of statistical knowledge among Cummins Inc.'s employees made teaching new Six Sigma trainees a challenge. Then the company tried Quality Trainer by Minitab, an e-learning course that teaches Green Belts, Black Belts and other quality professionals the statistics they need. Integrating Quality Trainer into their training program has helped Cummins roll out Six Sigma to more employees.
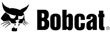
Bobcat
A quality expert at Bobcat used Minitab Statistical Software to increase the speed of a laser-cutting process while improving part quality, for a potential impact of more than $1 million.
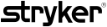
Stryker
Stryker, a leading medical technology company, credits their success to their culture of constant improvement. To make sure employees have the statistical knowledge they need, the company trusts Quality Trainer by Minitab, an e-learning course that teaches quality statistics and how to analyze data with Minitab Statistical Software.
.png)
OneSteel
When the amount of “mill scale” on steel rod from OneSteel’s Laverton Rod Mill began affecting yield and profitability at the company’s wire mill, the company used Six Sigma and the power of Minitab Statistical Software to find solutions—and demonstrate their success.
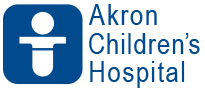
Akron Childrens Hospital
The largest pediatric health care provider in northeast Ohio uses quality techniques pioneered in industry to reduce patient waiting times and make many other improvements. And they trust Minitab Statistical Software to help them analyze their data and understand the results.
.png)
Mosaic Corporation
Find out how a Six Sigma team at Mosaic Corporation, armed with an excellent knowledge of process improvement and powerful tools including Minitab Statistical Software, increased productivity by more than 50 percent—and made a financial impact of more than $12 million per year.
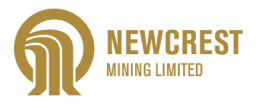
Newcrest Mining Ltd
With Minitab Statistical Software, Newcrest Mining can get an additional load of ore per truck each day from its Cadia Hill mine.
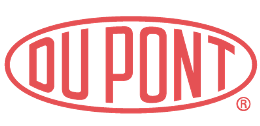
DuPont
DuPont Building Innovations reduced the amount of landfill waste they generated from 81 million pounds per year to zero. The global Six Sigma team that achieved this goal used Minitab Statistical Software to analyze and make sense of the tremendous amount of data gathered throughout the project.
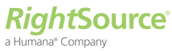
RightSource
When RightSource pharmacy implemented a new prescription management system, its productivity dropped and orders began backing up. Implementing a Lean Six Sigma project using Minitab allowed the company to reduce work-in-progress inventory by 40% and save money at every step.
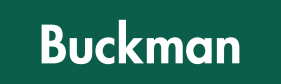
Buckman
Global chemical company Buckman transformed its business by training technical sales associates to use data analysis and Minitab to help customers improve their processes, provide more value to customers, and drive sales—impacting the bottom line.
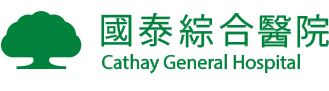
Cathay General Hospital
During an assessment of its angioplasty process for patients suffering from heart attacks, Cathay General Hospital in Taipei, Taiwan used Minitab Statistical Software to analyze data that would help save lives. The tools provided by Minitab allowed healthcare professionals to confidently introduce new treatment options that led to a decrease in the patients’ hospital stay and an increased savings in medical resources.
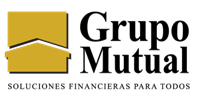
Grupo Mutual
A project team at Grupo Mutual, one of Costa Rica’s leading financial institutions sought to improve the cash flow among 55 of its bank branches. Using Lean Six Sigma methods and Minitab Statistical Software enabled the team to increase revenue by $1.1 million.

Edward Jones
With the current growth of the financial firm—and plans for future expansion—Edward Jones wanted to ensure its world-class service continues to exceed customers’ expectations. Armed with Lean Six Sigma tactics and the statistical tools in Minitab Statistical Software, the business process improvement team at Edward Jones set out to assess how the firm could continue to meet its high customer service standards in the midst of increasing service demands.
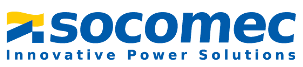
SOCOMEC
Energy supply specialist Socomec used Minitab Statistical Software and Lean Six Sigma methods to measure and improve its sales operations.
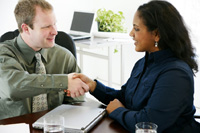
Human Resources
To investigate high employee attrition, the human resources department of a large manufacturer of aerospace engine parts conducted a Lean Six Sigma project and relied on the statistical tools in Minitab Statistical Software to analyze the data.
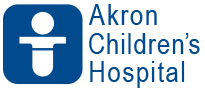
Akron Childrens Hospital
The largest pediatric health care provider in northeast Ohio uses quality techniques pioneered in industry to reduce patient waiting times and make many other improvements. And they trust Minitab Statistical Software to help them analyze their data and understand the results.