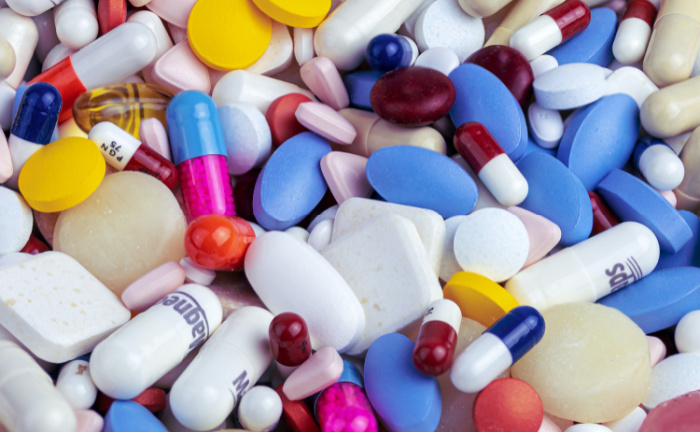
Based in Tokyo, Takeda Pharmaceutical Company Ltd. is a patient-focused, values-based, R&D-driven global biopharmaceutical company committed to bringing "Better Health and a Brighter Future" to people worldwide. Their passion and pursuit of potentially life-changing treatments for patients are deeply rooted in over 230 years of distinguished history in Japan.
Philippe Noquéro is a Europe Six Sigma Leader and a certified Master Black Belt (MBB) who is responsible for the Six Sigma capability across the Takeda network in Europe as part of the overall AGILE 4.0 program in place.
Takeda has had a longstanding relationship with Minitab and has used analysis to solve manufacturing issues, identify and execute on improvement opportunities, and design appropriate experiments to achieve process improvements with minimal supervision, among other things.
The Challenge
In Switzerland, Takeda Neuchâtel produces three drugs that treat two types of blood clotting disorders or deficiencies:
- Blood Disorder Type 1: Hemophilia A – these patients suffer from a missing or deficient amount of the VIII protein. Hemophilia A is the most common and well-known form of blood clotting disorders and deficiencies. Takeda produces these two clotting drugs for this disorder:
- Factor VIII: This is the protein required for the blood clotting chain
- Long-lasting Factor VIII: This is a long-acting version of Factor VIII in the patient’s body
- Blood Disorder Type 2: Von Willebrand disease (VWD) – this blood disorder does not allow the blood to clot properly. People with VWD either have a low level of the von Willebrand factor protein, or the protein does not work the way it should. Takeda produces one clotting drug for this disorder:
- Von Willebrand Factor: The protein needed for the blood clotting chain
The three drugs are also called "recombinant treatment," which means they are produced without the addition of human or animal derivatives. The drugs are derived from biotechnological production, using cell culture. As such, pharmaceutical products offer an extremely high degree of safety, purity, and efficacy.
Takeda wanted produce more of these drugs more efficiently, so it wanted to find a way to increase yield from the cell cultures used during the production process. To achieve this, the researchers needed to understand what process parameters affect cell culture performance.
The Solution
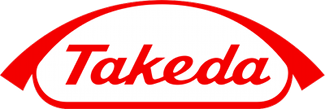
Customer
Takeda Pharmaceutical Company Limited
The Organization
- Founded 1781 Doshomachi, Osaka, Japan
- Traded on the NYSE (TAK)
- Largest pharmaceutical company in Asia
- Among top 20 largest pharma companies in world by revenue
The Challenge
Takeda wanted to produce more of three blood clotting drugs more efficiently, so it had to find a way to increase yield from the cell cultures used in production.
Products Used
Minitab® Statistical Software
Results
Researchers learned more about the yield process and were able to identify critical parameters.