Automation
Salford Predictive Modeler
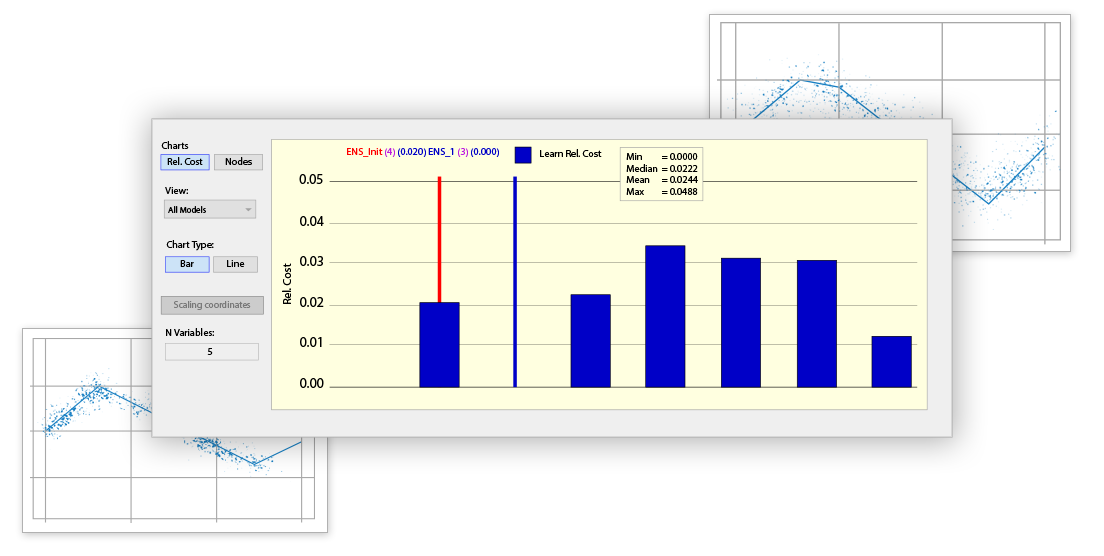
SPM® 8 comes with 70+ pre-packaged scenarios, basically experiments, inspired by how leading model analysts structure their work. We call them "Automates". These "Automates" or experiments create multiple models automatically so that the analyst can easily see choices.
Example 1: Banking Applications
Automate Shaving
Automate Shaving helps to identify subsets of informative data within large datasets containing correlated variables within the account data. With automation, you may accomplish significant model reduction with minimal (if any) sacrifice to model accuracy. For example, start with a complete list of variables, and run automated shaving from the top to eliminate variables that look promising on the learn sample but fail to generalize. Later you can run shaving from the bottom to automatically eliminate a major bulk of redundant and unnecessary predictors. Then follow up with "shaving error" to quickly zero in on the most informative subset of features.
As opposed to typical data mining tools, Automate Shaving offers more than the typical variable importance list. Additionally, the analyst is provided with a full set of variable importance subsets/variations enabling the analyst to quickly optimize/select the final variable list and eliminating the burden of repetitive testing. Expert modelers typically devote a lot of time and effort to optimizing their variable importance list; Automate Shaving automates this process.
Example 2: Fraud Detection
Automate Priors
In typical fraud detection applications the analyst is concerned with identifying different sets of rules leading to a varying probability of fraud. Decision trees and TreeNet® gradient boosting technology are typically used to build classification rules for detecting fraud. Any classification tree is constructed based on a specific user-supplied set of prior probabilities.
One set of priors will force trees to search for rules with high levels of fraud, while other sets of priors will produce trees with somewhat relaxed assumptions. To gain the most benefits of tree-based rule searching approaches, analysts will try a large number of different configurations of prior probabilities. This process is fully automated in Automate Priors. The result is a large collection of rules ranging from extremely high confidence fraud segments with low support to moderate indication of fraud segments with very wide support. For example, you can identify small segments with 100% fraud or you may find a large segment with a lesser probability of fraud, and everything in-between.
Example 3: Market Research - Surveys
Automate MVI (Missing Value Indicators)
In any survey, a large fraction of information may be missing. Often, the respondent will not answer questions either because they don't want to or are unable to do so. In addition to Salford Systems' expertise in handling missing values, a new automation feature allows the analyst to automatically generate multiple models including: 1) a model predicting response based solely on the pattern of missing values; 2) a model that automatically creates dummy missing value indicators in addition to the original set of predictors; and/or 3) a model that relies on engine-specific internal handling of missing values.
Example 4: Engineering Application
Automate Target
In a modern engineering application, as part of the experimental design, a large collection of sampled points may be gathered under different operating conditions. It can be challenging to identify mutual dependencies among the different parameters. For example, temperatures could be perfectly dependent on each other, or could be some unknown functions of other operating conditions like pressure and/or revolutions. Automate Target gives you powerful means to automatically explore and extract all mutual dependencies among predictors. By the word "dependencies," we mean a potentially nonlinear multivariate relationship that goes way beyond the simplicity of conventional correlations. Furthermore, as a powerful side effect, this Automate provides general means for missing value imputation, which is extremely useful to support those modeling engines that do not directly handle missing values.
Example 5: Web Advertising
Automate Sample
In an online ad placing application one has to balance the amount of data used vs. the time it takes to complete the model building. In web advertising there can be virtually an unlimited amount of data. So while ideally you would wish you use all available data, there is always a limit on how much can be used for real-time deployment. Automate Sample allows the analyst to automatically explore the impact of learn sample size on model accuracy. For example, you may discover that using 200,000,000 transactions provides no additional benefit in terms of model accuracy compared to 100,000,000 transactions.
Example 6: Microarray Application
Automate TARGETSHUFFLE
Microarray research datasets are characterized by an extremely large number of predictors (genes) and a very limited number of records (patients). This opens up a vast area of ambiguity resulting from the fact that even a random subset of predictors may produce a seemingly good looking model. Automate TARGETSHUFFLE allows you to determine whether the model performance is as accurate as it appears to be. Automate TARGETSHUFFLE automatically constructs a large number of auxiliary models based on randomly shuffled target variables. By comparing the actual model performance with the reference distribution (no dependency models), a final decision on model performance can be made. This technology could result in challenges to some of the currently produced papers in microarray research. If a dataset with deliberately destroyed target dependency can give you a model with good accuracy, then relying on the original model becomes rather dubious.
WHAT WE DO
Minitab products help businesses increase efficiency and
improve quality through smart data analysis.
Salford Predictive Modeler® 8
Minitab’s Integrated Suite of Machine Learning Software
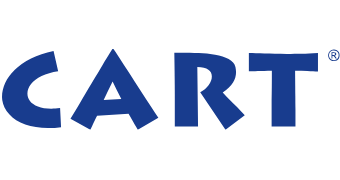
CART®
SPM’s CART® modeling engine is the ultimate classification tree that has revolutionized the field of advanced analytics, and inaugurated the current era of data science.
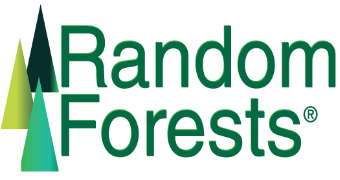
Random Forests®
Random Forests® is a modeling engine that leverages the power of multiple alternative analyses, randomization strategies, and ensemble learning.
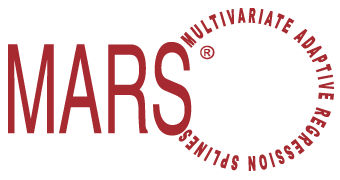
MARS®
The MARS® modeling engine is ideal for users who prefer results in a form similar to traditional regression while capturing essential nonlinearities and interactions.
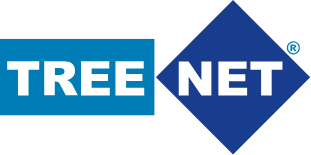
TreeNet®
TreeNet® Gradient Boosting is SPM’s most flexible and powerful data mining tool, capable of consistently generating extremely accurate models.
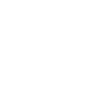
Pricing
Contact us for pricing information.
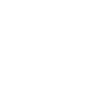
University Program
Our University Program provides the SPM®, CART®, MARS®, TreeNet® , and Random Forests® modeling engines at significantly-reduced licensing fees to the educational community.
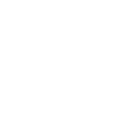
Automation
70+ pre-packaged scenarios, basically experiments, inspired by how leading model analysts structure their work.