Data-driven process improvement initiatives are typically associated with industries like manufacturing. However, the process of using statistics and data analysis to solve problems is flexible enough to benefit any business function. "Lean Six Sigma has grown in transactional and service-based environments," says Jeff Parks, Lean Six Sigma master black belt. "For example, I’ve had the opportunity to apply quality improvement techniques to the area of human resources." In one recent Lean Six Sigma project, Parks set out to understand and reduce high employee attrition at a large manufacturer of aerospace engine parts, relying on the powerful tools in Minitab Statistical Software to analyze the data.
The Challenge
Employee attrition, or turnover, is the rate at which workers leave a company. Because of the expenses associated with hiring and training new employees, high attrition rates can be problematic—and very costly—for companies.
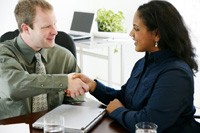
Jeff Parks, a Lean Six Sigma master black belt, used Minitab Statistical Software to solve a key human resources problem for a major manufacturer of aerospace engine parts.
In 2009, a large Ohio manufacturer of aerospace engine parts had normal attrition rates of 15-18 percent, which mirrored general manufacturing attrition rates for the region where the company was based. However, the downturn of the U.S. economy in 2009 coincided with an increase in the company’s attrition rate, which soared to more than 30 percent. Given the high unemployment rates regionally and nationwide, management expected the rates to be much lower. "The high attrition rate was very problematic," says Parks, who led the company’s quality improvement efforts. "It implied a deeper problem existed within the company—one that the human resources department couldn’t wrap their arms around."
Human resources personnel at the manufacturer typically relied on exit interviews to understand why employees leave. While a lot can be learned from these interviews, there is no opportunity to conduct an exit interview when employees leave without warning. And in an hourly manufacturing environment where the work is very laborious, it was not uncommon for employees to quit unannounced, leaving behind little or no information about what prompted their departure. The manufacturer had compiled basic data about employees who had left their jobs unannounced over the past two years, and Parks took on the task of analyzing the data to see what insights it might offer about the sudden rise in attrition.
How Minitab Helped
The company had hired 100 employees over a span of two years. Of those new hires, 32 had quit unannounced. The company had basic information about each of the new hires, including the employee’s gender, position, pay classification, shift worked, prior years of manufacturing experience, and length of commute. To see if statistically significant differences existed between the proportion of employees who quit across pay classifications (hourly vs. salary) and shifts worked (first shift vs. second shift), Parks performed hypothesis tests in Minitab. He had hoped to reveal patterns in employee attrition behavior, but the analysis showed no statistically significant differences between the proportions for any of the variables.
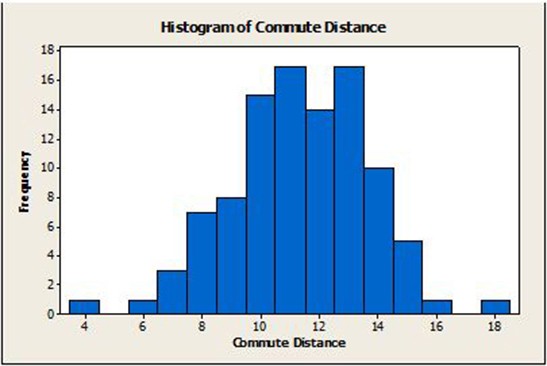
With Minitab charts, Parks was able to visualize raw data the company compiled about employees with attrition behavior. The histogram above outlines the distribution of commute distances for newly hired employees over the course of two years.
But Parks didn’t stop there. He investigated the raw data further, and noticed that many of the employees who quit were females with prior manufacturing experience who traveled various distances to work. He used Minitab to find out if gender, prior years of manufacturing experience, or commuting distance correlated with whether or not the employee had left the company. In this case, the response Parks wanted to assess—quitting or not quitting—was binary and only had two possible values. Minitab’s powerful binary logistic regression analysis allowed Parks to create statistical models to predict which variables might have made a person more likely to quit.
The analysis revealed that the length of an employee’s commute was statistically significant. The output from his Minitab analysis yielded a regression equation Parks could use to predict the probability of employees quitting based on the number of miles that made up their commute to work. He used the equation to analyze distances up to 30 miles, and found that commuting distance had little impact on the probability of an employee quitting until the 12 mile mark. At 12 miles, the probability of an employee quitting increased to more than 18 percent. "And at 13 miles, which is about a 30-45 minute commute, the probability of quitting jumped to more than 92 percent," Parks notes. "If the commute exceeds 13 miles, it is almost assured that an employee will quit."
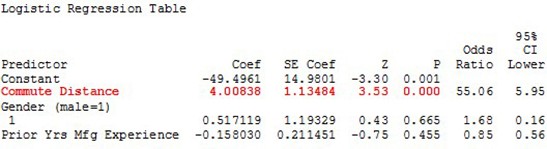
Minitab’s powerful logistic regression analysis helped Parks pinpoint the factors that correlated with employees who quit their jobs unannounced.
The Results
The manufacturer’s HR department used the results of Parks’ analysis to revamp the interview process for manufacturing positions. They began to look more closely at the potential employee’s commuting distance and took this into account before making hiring decisions.
Parks believes the data explain the seeming paradox between a rising attrition rate and a time of high unemployment. "The analysis seemed to imply that during the recession, people were very liberal about the jobs they applied for because the economy was so bad," says Parks. "Once employees realized that the commute could be arduous, they quickly went on to look for other jobs, and often would quit."
Parks has been a Minitab user for more than 15 years and likes that the software can be used by people of all skill levels and from various backgrounds. "Minitab has allowed me to teach hundreds of belts with limited statistical ability, and complete quality improvement projects across many different business areas," he says. "In terms of ease-of-use and statistical power, it’s the best statistical package on the market."
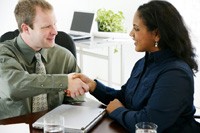
Overview
- Major Ohio manufacturer of aerospace engine parts
- Attrition rate for manufacturing positions increased significantly during the economic downturn
- Reliance on exit interviews to assess why employees leave couldn’t account for workers who quit unannounced
Challenge
Understand the factors affecting employee attrition rates.
Products Used
Minitab® Statistical Software
Results
- Gained insight about employees who quit unannounced
- Commute distance became a factor to consider when making hiring decisions
- Enhanced the interview process